Exploring Failed Case Studies on the Use of AI in Denial Management in Clinical Diagnostic Labs
In recent years, the use of Artificial Intelligence (AI) in various industries has been on the rise, including within clinical Diagnostic Labs. AI has the potential to improve efficiency, accuracy, and overall Workflow in these labs. One area where AI has been explored is in denial management, which involves identifying and resolving issues related to claims denials from insurance companies. While there have been successful case studies of AI implementation in denial management, there have also been instances where AI has failed to deliver the expected results. In this blog post, we will explore some of the failed case studies regarding the use of AI in denial management within clinical Diagnostic Labs.
Background
Before diving into specific case studies, it's important to understand the role of denial management in clinical Diagnostic Labs. When a claim is denied by an insurance company, it can result in delays in payment and financial losses for the lab. Denial management involves identifying the reasons for denials, resolving them, and resubmitting claims to ensure timely payment. AI has been touted as a tool that can streamline this process by identifying patterns in denials, predicting potential issues, and providing recommendations for resolution.
Failed Case Studies
Case Study 1: Inaccurate Prediction Models
One of the common reasons for failures in AI implementation in denial management is inaccurate prediction models. In one case study, a clinical diagnostic lab implemented an AI system to predict the likelihood of claims denials based on historical data. However, the AI system consistently generated inaccurate predictions, leading to incorrect prioritization of denial resolution efforts. This resulted in delays in payments and increased financial losses for the lab.
Despite efforts to refine the AI algorithms and retrain the system, the inaccuracies persisted, ultimately leading to the abandonment of the AI system for denial management. This case study highlights the importance of ensuring the accuracy and reliability of prediction models before implementing AI in denial management.
Case Study 2: Lack of Integration with Existing Systems
Another common pitfall in AI implementation in denial management is the lack of integration with existing systems. In a different case study, a clinical diagnostic lab invested in an AI tool to streamline denial management processes. However, the AI system was not compatible with the lab's existing billing and claims management systems, making it difficult to integrate and utilize effectively.
As a result, the lab faced challenges in accessing and analyzing data, communicating with insurance companies, and resolving denials in a timely manner. Despite attempts to bridge the gap between the AI system and existing systems, the lack of seamless integration ultimately led to the failure of the AI implementation in denial management.
Case Study 3: Inadequate Training and Support
In some cases, the failure of AI implementation in denial management can be attributed to inadequate training and support for end-users. In a particular case study, a clinical diagnostic lab adopted an AI tool for identifying patterns in claims denials and recommending resolution strategies. However, the lab staff did not receive sufficient training on how to effectively use the AI system or interpret its recommendations.
As a result, the staff struggled to navigate the AI interface, understand the insights generated by the system, and implement recommended strategies for denial resolution. This lack of training and support for end-users led to frustration, resistance to using the AI tool, and ultimately, the abandonment of the system for denial management.
Lessons Learned
While the aforementioned case studies highlight the challenges and failures of AI implementation in denial management within clinical Diagnostic Labs, there are valuable lessons to be learned from these experiences. Some key takeaways include:
Ensuring the accuracy and reliability of AI prediction models before implementation
Ensuring seamless integration of AI systems with existing infrastructure and systems
Providing adequate training and support for end-users to effectively utilize AI tools
Regularly monitoring and evaluating the performance of AI systems in denial management
Conclusion
While AI holds great promise for improving denial management processes within clinical Diagnostic Labs, there have been instances of failed case studies where AI systems did not deliver the expected results. By understanding the common pitfalls and lessons learned from these failures, labs can better navigate the implementation of AI in denial management and optimize its benefits in improving efficiency, accuracy, and financial outcomes.
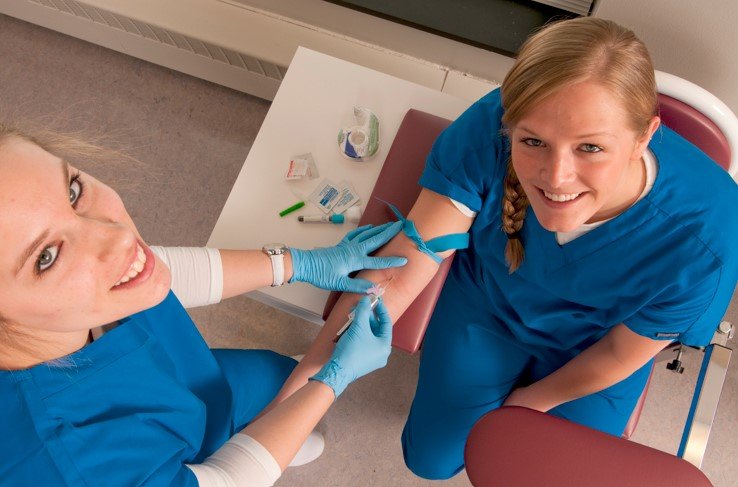
Related Videos
Disclaimer: The content provided on this blog is for informational purposes only, reflecting the personal opinions and insights of the author(s) on phlebotomy practices and healthcare. The information provided should not be used for diagnosing or treating a health problem or disease, and those seeking personal medical advice should consult with a licensed physician. Always seek the advice of your doctor or other qualified health provider regarding a medical condition. Never disregard professional medical advice or delay in seeking it because of something you have read on this website. If you think you may have a medical emergency, call 911 or go to the nearest emergency room immediately. No physician-patient relationship is created by this web site or its use. No contributors to this web site make any representations, express or implied, with respect to the information provided herein or to its use. While we strive to share accurate and up-to-date information, we cannot guarantee the completeness, reliability, or accuracy of the content. The blog may also include links to external websites and resources for the convenience of our readers. Please note that linking to other sites does not imply endorsement of their content, practices, or services by us. Readers should use their discretion and judgment while exploring any external links and resources mentioned on this blog.